For organizations of all sizes, AI implementation should never resume to just adopting the latest AI technologies. Like any innovation, it comes with measuring effectiveness and responsible deployment.
On one hand, you have the operational side: AI can streamline processes, drive efficiencies, and deliver powerful insights. But on the other hand, one can’t ignore the ethical implications. How we design, deploy, and manage AI systems impacts society, privacy, fairness, and transparency.
While AI applications are becoming more evident each day, there’s still an acute lack of know-how regarding compliant AI implementation, which is the central theme of this article.
Let’s dive into a proven 7-step approach to implementing AI effectively while adhering to regulatory considerations.
Zitec’s 7-Step Roadmap to Successfully Implement AI
To guide companies through the complexities of AI adoption, we’ve developed a structured roadmap for successful AI implementation. This map serves the purpose of aligning leaders in innovation with business goals.
1. Define clear business objectives
AI initiatives should never be an afterthought or a random experiment. It must be aligned with specific business goals to deliver real value. Before implementation, clearly define what success looks like: Are you aiming for:
- Cost reduction
- Increased efficiency
- Improved customer engagement
- Any other goals specific to your business?
For example, according to McKinsey, AI-powered automation can reduce operational costs by up to 40% in customer service, especially when focused on high-impact tasks like handling routine inquiries. Could this be a priority area for your organization? |
To form a solid strategy and define business objectives around AI adoption, consider gathering employee insights to identify inefficiencies. Often, the frontline staff will pinpoint areas that AI can solve effectively. During this stage, employee workshops or surveys are great ways to collect insights.
Remember that not all business processes needs AI. Some tasks can be efficiently handled through simple automation. The key is to prioritize the right business scenarios and use cases with the highest potential business impact, where AI can deliver actual value.
For example, automating invoicing or inventory management might be more immediately impactful than experimenting with AI-driven sales predictions. However, it all ties back to your goals and the type of investment you’re aiming for. |
2. Assess organizational readiness
AI implementation starts with a comprehensive readiness assessment across data, technology, skills, and culture:
Data quality and readiness
AI thrives on high-quality, accessible, and secure data, which might be easier said than achieved. According to a 2024 Accenture report, 61% of organizations report that their data assets are not ready for generative AI – but there’s a way to change that. Start by evaluating your data quality and infrastructure: Is it accurate, clean, and well-organized?
Organizations often overlook the vast amounts of unused or incomplete data they already possess. These "data mountains" can be a goldmine, but only if they are properly structured and integrated.
In eCommerce, businesses often deal with vast amounts of both structured and unstructured data, which are invaluable for building customer experience solutions. Scalable, modern platforms are key to harnessing this data, as they allow for the use of AI tools like predictive analytics, machine learning, and automated decision-making business processes.
Technology audit
Can your current systems integrate seamlessly with AI applications? For instance, if you're using legacy systems for your CRM, assess their compatibility with AI-driven solutions using models like predictive analytics or machine learning or off-the-shelf AI tools. Identify any infrastructure gaps, such as data storage or computing power, that could slow down or block AI deployment.
Nonetheless, it’s crucial to invest in scalable cloud solutions if you anticipate growth in data volume or processing needs, or if your legacy systems are struggling to keep up with the computing power required to achieve the utmost mode performance or simply keep up operations that will become AI-dependent.
Skill assessment
AI is complex, and your internal teams may need upskilling. Start by evaluating whether your in-house team has the expertise to manage AI projects, from data scientists to AI engineers. If gaps exist, consider partnering with technological leaders with a proven track record, who have the expertise to guide you through implementing AI strategies without disrupting business operations.
Cultural alignment
Employees must not see new AI tools as a replacement, but as an amplifier of their productivity and creativity. Encourage an environment where innovation is fostered and AI’s potential is clearly communicated.
3. Understand the basics of AI
Here, the question isn’t “What can AI do?” but rather, “How can AI drive real value for my business today?” So far, third-party data show a clear trend – the tangible benefits are evident, as long as goals and processes align strategically.
Naturally, just like with every investment, not all AI experiments end in successful deployment and transformative outcomes. That’s why a strategic approach to objective setting and AI implementation can help maximize the value of this technology.
McKinsey suggests that Gen AI can automate work activities that take up as much as 60%-70% of an employee’s time today. In industries like retail, the measured ROI adds a margin of 1.2 to 1.9%. |
According to the same company, approximately 75% of the value that generative AI use cases could deliver falls into four types of solutions:
- Customer operations
- Marketing and sales
- Software engineering
- R&D
Mapping this to our very own use cases, we can see that the findings align. Here’s where we see implementing AI delivering the most business value:
Automation for time and cost-optimization
AI thrives on eliminating repetitive tasks, allowing your team to focus on higher-value work. Think about how much time your organization spends on data entry or routine analysis—AI can take that off your plate.
Let’s consider the example of our Ministry of Environment project, where implementing AI automated processes that once required extensive human resources for oversight, proving it could cut manual labor by 99% and reduce costs by 97%. That’s not just optimization — that’s quite literally digital transformation. Read more about our partnership with the Ministry (Romanian). |
This type of automation allows your people to work smarter. By freeing up resources, implementing artificial intelligence allows teams to prioritize strategy, innovation, and growth.
Real-time data processing for smarter decisions
AI in retail, for example, is known for analyzing live data, such as consumer demand, seasonal trends, and competitor pricing, and then dynamically adjusting pricing strategies or inventory levels. This kind of agility wasn’t possible with traditional monthly or quarterly reporting cycles.
But the real power lies in AI’s ability to forward decision-making. Imagine implementing AI to identify untapped market segments or perform a SWOT analysis at scale. Rather than reacting to changes, AI tools and custom AI solutions offer the ability to predict them and act first.
Customer personalization at scale
Customers expect tailored experiences, and AI makes that scalable. By analyzing behaviors, preferences, and purchase histories, AI delivers hyper-personalized recommendations.
In our work with e-commerce clients such as FashionDays, AI-powered recommendation engines increased conversion rates and drove customer loyalty by suggesting products customers didn’t even know they wanted in the first place |
Here’s an example: AI notices a customer consistently buys eco-friendly products and recommends complementary items. AI tools like Google’s RecommendationsAI take this even further by automating and refining these suggestions at scale. Beyond individual personalization, AI identifies new opportunities, like what products perform best in different regions, which helps businesses expand with confidence.
4. Evaluate potential ROI
By now, you’ve clarified your objectives, assessed your organizational readiness, and understood how AI implementation can deliver business value. The next step? Ensuring your investment pays off. This means validating AI’s potential through a Proof of Concept (PoC) before committing at scale.
Start with high-value use cases
Your PoC should focus on areas where AI aligns directly with your business goals. For instance, if cost reduction is your priority, target repetitive, time-consuming processes like inventory forecasting or customer inquiry handling.
Test in real-world conditions
A PoC gives you the ability to test AI in real-world scenarios, not theoretical ones. If your goal is to improve customer engagement, start by piloting a chatbot for one customer segment and measure its impact on response times or satisfaction scores. Or, in e-commerce, you can test an AI-driven recommendation engine on a subset of products or customers to measure its effect on conversions.
Define and measure ROI metrics
Source: Zitec Client Case Study Results
AI’s ROI is multi-dimensional. While financial outcomes like cost savings or revenue growth are obvious metrics, consider the intangible benefits of implementing AI as well. These might include:
- employee efficiency
- customer satisfaction
- time-to-market for decisions
One of the most overlooked aspects of a PoC is its role in providing actionable learnings. If your PoC highlights unexpected challenges, like gaps in your data infrastructure or unforeseen user resistance, that should be considered a good return on investment.
A well-executed PoC isn’t just a testing phase, but a tool for stakeholder buy-in. Present measurable outcomes that align with your defined objectives, like reducing average customer response times or operational costs by a specific amount. When stakeholders see clear, data-backed results, it’s easier to secure resources and commitment for broader AI integration and adoption.
5. Choose the right AI solution
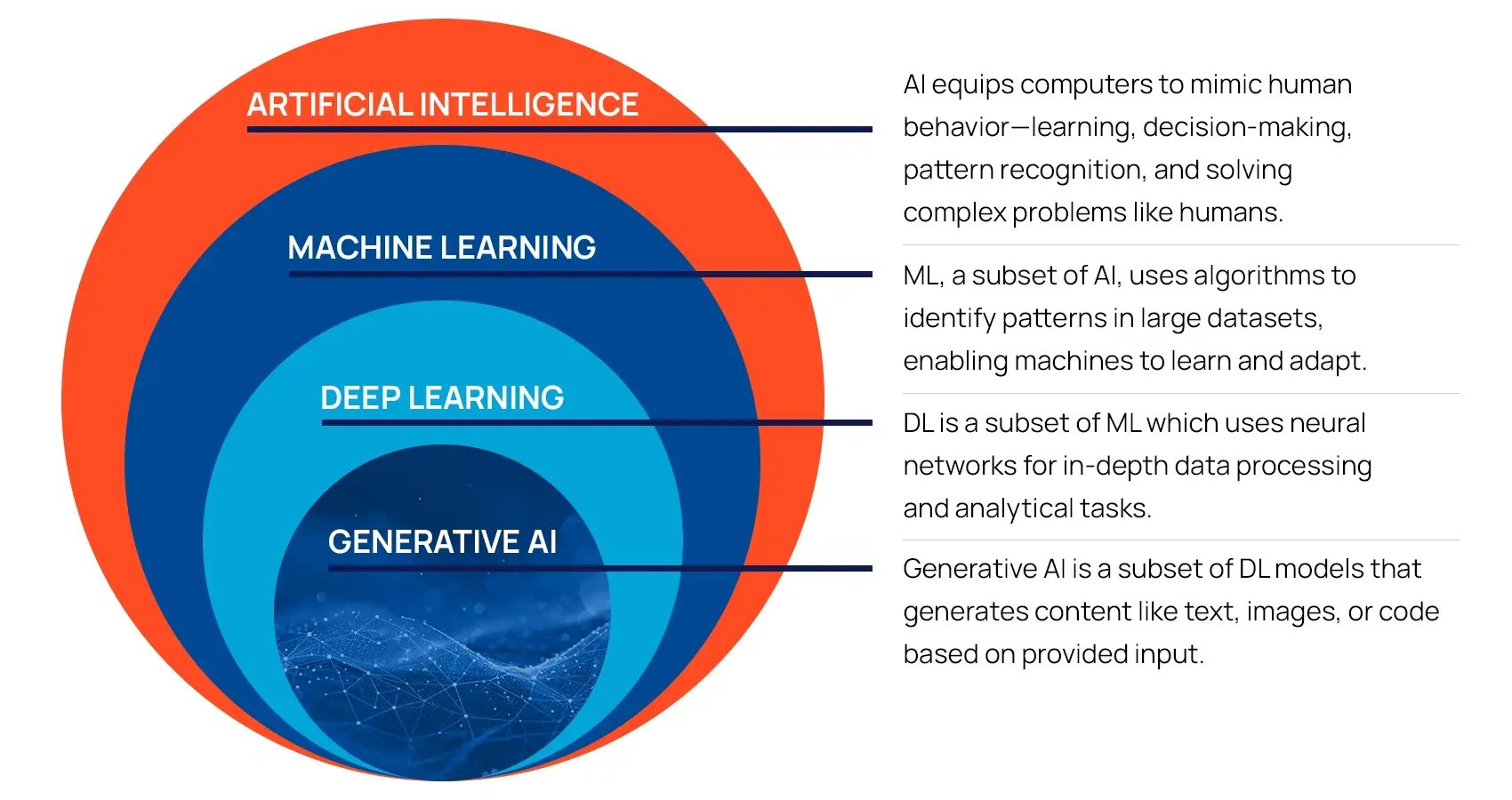
Source
This part is not about opting for the most advanced technology, but about aligning the solution with your goals, data, and existing infrastructure, and matching the complexity of the right AI model to the scope of the problem.
AI solutions range from Natural Language Processing (NLP) for chatbots and sentiment analysis to Machine Learning (ML), Predictive Analytics, and Deep Learning for complex tasks. Other options of an AI model, such as Generative AI for personalized content or Reinforcement Learning for decision-making, cater to niche challenges.
Choosing the right solution and AI model requires understanding its fit for your business goals. If internal expertise is limited, a strategic collaboration with an experienced AI partner is crucial for a well-defined strategy and successful integration. Look for partners with industry-specific knowledge who can adapt solutions to your business context instead of offering generic off-the-shelf patches to your needs.
Evaluate potential partners based on:
- Proven success in similar industries.
- Transparent communication and adaptability.
- Scalability of their solutions to meet evolving needs.
Ready to explore AI solutions tailored to your business? Our advice is to start small. 👇
6. Communicate and train
When it comes to implementing AI either via AI tools, custom solutions, or an AI integration, the human factor is just as critical as the technology itself. The first step in a successful AI rollout is effective communication. Employees need to understand the why behind the change, or how AI will empower them instead of replacing them.
AI should be positioned as a tool that amplifies their expertise, not one that diminishes it, to overcome natural resistance to new technologies. Just as stakeholders require nurturing and transparent communication before complete buy-in, so do employees.
Share clear, realistic expectations about how AI will be integrated into workflows, and involve employees early in the process to get their buy-in. On the training front, a one-size-fits-all approach won’t work, meaning:
- Frontline employees require hands-on sessions so that they get comfortable with the AI tools they’ll interact with daily.
- Leadership and top management will benefit from workshops that explore the strategic implications of AI for the business.
Furthermore, AI training shouldn't be a one-off event. To incorporate AI into all relevant aspects of your organization, understand that this is an ongoing process aimed at empowering people to adapt to evolving technology.
7. Establish KPIs and monitor success
The key to setting realistic, actionable KPIs for AI is making sure that they’re tied to the business outcomes that matter most. Whether that’s cost savings, customer satisfaction, or revenue growth, KPIs will look different, but they should all follow a SMART structure.
Let’s discuss an example of boosting productivity as a KPI, measured in %, tied to the bigger organizational goal of boosting operational efficiency:
|
EU’s AI Act – How to Implement AI through Responsible Innovation
Whether we like it or not, ethically implementing AI is a business priority, particularly as regulations evolve globally. The European Union has taken the lead in this sphere by introducing the EU AI Act, the first comprehensive legal framework for AI deployment.
The EU AI Act classifies AI systems based on the level of risk they pose. AI systems are divided into four categories, ranging from minimal to unacceptable risks. The higher the risk, the stricter the regulations.
Source
For instance, AI systems that involve social scoring or exploit vulnerable groups are prohibited. High-risk systems, such as facial recognition or AI used in hiring decisions, require:
- Rigorous oversight
- Including risk assessments
- High-quality datasets to minimize bias
- Clear documentation for authorities
Naturally, transparency is a cornerstone of responsible AI. For example, AI systems with limited risk (such as chatbots) must inform users that they are interacting with a machine. Additionally, businesses must establish traceability for high-risk systems through activity logging and documentation, to make it easy to audit AI’s decisions. Ultimately, it all comes down to the degree to which AI is used, the operations it covers, and the data fed in the model training process.
When we integrate AI, we strive to push the boundaries of innovation responsibility. Ethical guidelines shape how we design, deploy, and maintain our AI systems so that they’re transparent and accountable. We treat regulatory guidelines not as another checkbox, but as a compliant pathway to ROI. That’s how our public sector project blended responsible data use with proven operational efficiency, which translated into time savings of over 99%. For Zitec, delivering this solution for the Ministry of Environment was tangible proof that ESG, ethical data governance, and AI truly go hand-in-hand. We're excited to replicate this success and apply our learnings in the area of data & AI.
Data & AI Director, Zitec
Since AI systems rely heavily on data, adhering to the EU's General Data Protection Regulation (GDPR) is non-negotiable. Companies must obtain explicit consent before collecting personal data and guarantee that data is secured through encryption, access controls, and regular audits to prevent breaches.
Beyond legal compliance, ethical considerations, such as bias and fairness must be considered. AI models can unintentionally perpetuate discrimination if trained on biased data. Regular audits, diverse datasets, and transparency in decision-making can help mitigate this risk.
Frequently Asked Questions (FAQs)
The process begins with defining clear goals to ensure AI addresses specific business challenges directly. Following this, an assessment of data quality, infrastructure, and skill sets determines organizational readiness. Identifying high-impact areas allows businesses to focus AI efforts where they’ll yield the most value. Selecting AI solutions tailored to data requirements and problem complexity is key, and a proof-of-concept phase tests effectiveness before broader deployment. Integration into operations is done smoothly to minimize disruptions, with ongoing monitoring and refinement ensuring that AI continues to drive measurable results.
AI implementation costs can vary significantly depending on factors like project scope, data complexity, and model sophistication. For instance, simpler projects like chatbots or automated data analysis typically incur lower costs compared to more complex systems, such as large-scale machine learning models or predictive analytics. To manage costs effectively, adopting a Proof of Concept (PoC) approach can be invaluable. By testing AI on a smaller scale first, businesses can identify potential challenges, refine the model, and adjust the budget before committing to full-scale deployment. Partnering with experienced technology solutions providers is crucial, as they can help define realistic budgets, optimize resource allocation, and ensure cost-efficiency while aligning the AI solution with overall business goals.
Generative AI (Gen AI) is a type of artificial intelligence that creates new content, such as text, images, code, or audio, based on patterns learned from existing data. These systems, like those used in content creation and data augmentation, bring unique capabilities to businesses, allowing them to automate and improve creative processes. When implementing Generative AI, it’s essential to address data security and bias mitigation to ensure ethical use. Additionally, human oversight is recommended to verify outputs, as Generative AI can sometimes produce unexpected or inaccurate results.