People like recommendations. Whether you’re looking for someone to replace bathroom tile, or you’re shopping for a new child car seat, you feel more confident when you receive a recommendation from a trusted source. The same goes for online shopping.
Online sales are predicted to grow by 50% in the next four years. It is a staggering rate and signs of changing behaviors are visible all around us. From the digital native Gen Z to the late adopting seniors of the world, online shopping has become the main choice for a wide range of items, replacing physical stores as a primary option in many areas. On the one hand, there has never been a better time to start a new ecommerce business. On the other, it can be particularly frightening for many customers to go diving into the sea of diversity that is the internet. This is when having the suitable recommendation at the right time becomes invaluable.
For eCommerce businesses, personalizing the customer journey, achieving high levels of customer satisfaction, and creating loyalty are high on the priority list. But many struggle to get it right. People return to the same online stores because they feel familiar and friendly, just like the brick-and-mortar places they used to visit.
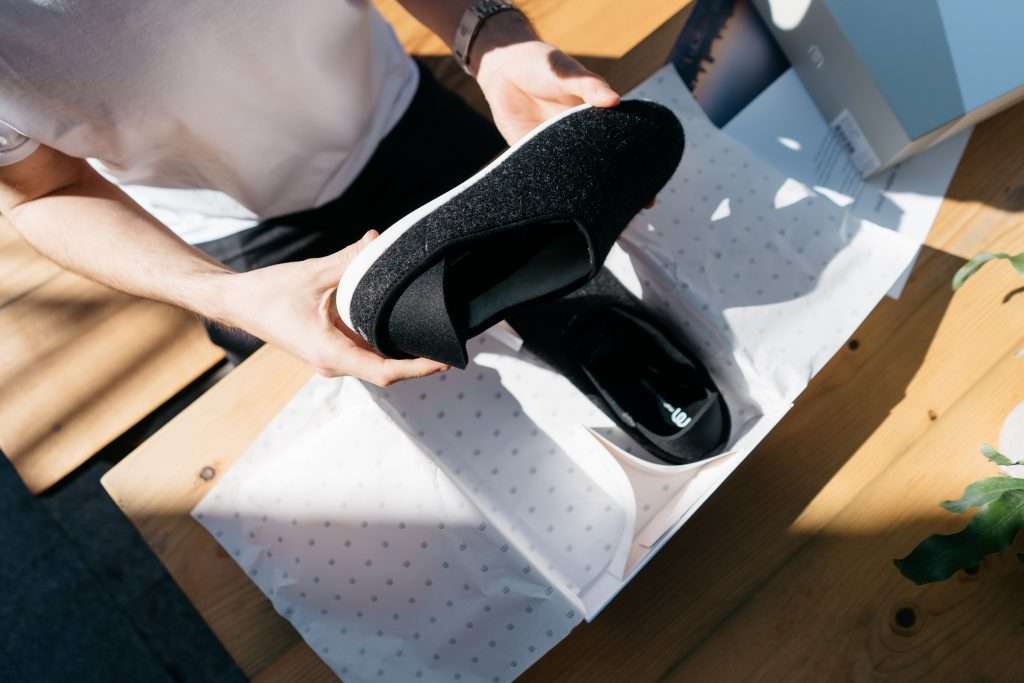
One of the best ways to create this kind of experience is through recommendations. There are several ways to implement AI powered recommendation models. Some companies take the in-house route. While it can produce good results for basket growth and revenue, it tends to be the less resource-friendly alternative. Having dedicated staff to manually curate rules and manage difficult recommendation models can be costly and less time efficient.
Then there is Google’s Recommendations AI. In a previous article we covered how Google’s Recommendations AI can help online companies grow by delivering a seamless, enjoyable shopping experience. But how is it better than having your staff team create dedicated recommendation models? The short answer is Google itself. Recommendations AI is powered by the same technology behind Google Search and YouTube. Its deep learning models are context hungry, leveraging both item and user metadata to draw insights across millions of items. Achieving this through manually curated rules would be an almost impossible task. It also eliminates the need to write thousands of lines of code to train custom recommendation models and keep them up to date with variables.
Recommendation types
Recommendations AI provides ecommerce websites with four types of recommendations.
· Others you may like
– Predicts the next product that a user is most likely to engage or convert
– Based on the user’s shopping and viewing history and the relevance of a candidate product compared to the current specific product
· Frequently bought together
– Predicts items frequently purchased together in the same session
– If a list of items is purchased, the model also forecasts products bought together with that list
– It is useful when the user has indicated the intention to buy a particular item and the seller wants to recommend complementary articles It is commonly displayed in the “Add to Cart” page
· Recommended for you
– Predicts the next product the user is most likely to engage with or buy, based on shopping and viewing history
– Typically used on the homepage
– Can also be used on category pages
· Recently viewed
– Not actually a recommendation
– Provides the IDs of products the user has recently interacted with, displaying the most recent interaction first
Got something specific in mind? Optimize!
One of the great parts about machine learning models is that you can optimize them to serve your specific goals. Each model has a default optimization; however, this is not a limitation by any means. Depending on your unique goals, our Google Recommendations AI team can create a different optimization objective, more suited to your target achievements. Once the model has been trained, the objective cannot be changed, so each new objective will require a separate optimization. Recommendations AI has several optimization objective templates, ready to go. Here is a quick overview.
Click Through Rate, CTR
– Emphasizes engagement; best used when the intention is to maximize the chances of the user interacting with the recommendation
– It is the default optimization for the “Others you may like” and “Recommended for you” models.
Revenue per order
– Cannot be used with any other model
– It is the default optimization for “Frequently bought together” model
Conversion Rate, CVR
– It maximizes the chances for users to add recommended items to their cart
– Ideal for increasing the number of items added to the cart per session
Recommendations AI also has several advanced configuration models available for customization by certified experts. Zitec’s Recommendations AI team has been helping online companies reach their target goals by implementing and customizing recommendation models. Reading this far is a good indication that you want your clients to have the best possible shopping experience on your website. It might have also left you with several question marks. Whichever the case, let our Recommendations AI team know what’s on your mind.